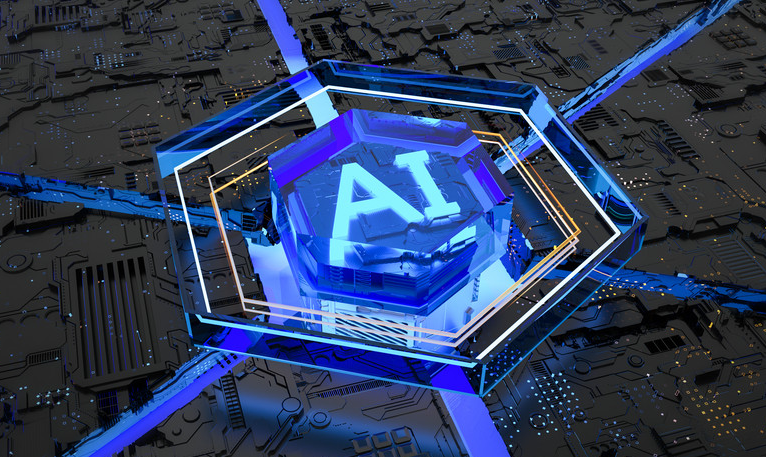
According to statistics, the security threat caused by identity fraud continues to grow rapidly. Last year alone, corporate organizations in the United States lost more than $20 billion in financial losses due to identity fraud, with an identity fraud occurring every 14 seconds. Among them, synthetic identity fraud is the fastest growing identity fraud model. Cyber attackers integrate fake information data into some real user information to generate fake or synthetic identities, which poses a serious threat to traditional fraud detection models.
As identity fraud models evolve, driven by huge business interests, fraud threat analysts are exhausted. Due to the lack of real-time visibility of existing fraud detection models, it is difficult to achieve comprehensive analysis of extensive monitoring data. As a result, the existing fraud prevention model technology, BAR43S, cannot build new security boundaries for organizations through authentication. For organizations, there is a need to deploy more effective applications and tools as quickly as possible to combat the growing threat of identity.
While improving the identification rate of identity fraud verification, it is not easy to reduce the false positive rate. Identity security manufacturers are trying to use different identity security manufacturers in different ways, and AI technology and machine learning models achieve this goal, but the technical concept is basically the same, that is, through continuous model training and setting of trustscore based on business scenarios, combined with structured and unstructured machine learning. Quickly match appropriate risk assessment scores to verify the legitimacy and security of new accounts.
In order to better address the threat of identity fraud, security analysts need to be provided with more insightful intelligent analytics tools to identify more potential identity fraud risks based on constraint rules. This requires better training and supervision of machine learning algorithms through AI automation to identify anomalies that cannot be detected by existing fraud detection techniques, complemented by unsupervised machine learning techniques to explore new patterns of suspicious identity discovery. Here are five scenarios where AI technology can help detect identity fraud:
1. Make identity control policies more reliable
In order to promote business development, customers usually prioritize online services, do a good job of authentication and identification, and effectively protect the privacy of customers. But the reality is that synthetic fraud can avoid most fraud prevention techniques without guaranteeing an identity trust score. Because without long-term analysis based on transactions, combined with real-time trusted identity management and trusted identity models, it is difficult to provide an accurate assessment of identity trust.
Through the use of AI technology, account trust and user experience (UX) effective combination to make the enterprise identity control strategy really work. By efficient combination. AI systems assess the trustworthiness of identity accounts in real time, helping to prevent fraud analysts from creating more effective rules and workflows based on constraints, saving time and improving detection efficiency.
2. Realize the coordination ability of identity proof, fraud detection and user identity verification
Currently, the three strategies of authentication, fraud detection, and user authentication are often isolated from each other. Need it now. Only by providing contextual intelligence can an organization fully understand all the risks that customer entities face. Working on fraud detection means it will be a fraud detection. AI analytics capabilities are integrated into the overall technical architecture of fraud identification.
To improve the accuracy of identity fraud, fraud detection, and user verification, we need to break down the barriers between different systems. To successfully combat synthetic fraud, the organization needed an integrated end-to-end platform. The richer the data that can be analyzed, the more representative it is, and the more likely it is that synthetic identity fraud will be detected. JimCunha, head of Secure payments Strategy at the Federal Reserve Bank of Boston, said: "Organizations are more likely to identify new identity fraud if they employ a layered fraud mitigation approach that combines manual data analytics with technical data analytics. In addition, internal information sharing and information sharing with other parts of the payments industry can help organizations understand the changing technology of identity fraud."
3. Discover abnormal behaviors based on your identity in real time
The identity-based transaction data training model can provide a real-time risk score for each transaction and identify whether the transaction behavior is abnormal, so as to identify identity fraud more targeted. When evaluating fraud detection platforms, companies should look for solutions that combine supervised and unsupervised machine learning techniques to improve the recognition of unusual behavior. At present, some advanced fraud prevention and recognition verification platforms can dynamically build convolutional neural networks and continue to do deeper recognition "learning" from the real-time recognition data of machine learning algorithms.
4. Help zero trust technology apply to fraud prevention platforms
A good zero trust strategy is critical to reducing and eliminating identity fraud. The core concept of zero trust should be a fundamental requirement for effective fraud prevention strategies. Enterprises should start with multi-factor authentication and selectively apply AI machine learning to address complex challenges.
If the core principles of zero trust (including least privileged access, identity and access management, micro-segmentation, and privileged access management) are supported by AI, the success of fraud will rapidly diminish. Identity has become the most common threat exposure, and organizations need to make intelligent authentication one of the basic jobs of a zero-trust framework.
5. Scientifically optimize protection strategies to ensure business continuity
False positives are one of the main causes of digital service disruption. One of the contradictions that identity fraud analysts often face is that identity control strategies are set up not only to prevent fraud losses, but also to guarantee the normal development of digital business. Fraud analysts cannot set emotional protection strategies based on their own feelings, but can seek to help AI strategy optimization methods based on supervised and unsupervised learning abilities. In addition, the AI-based fraud recognition rate model can effectively reduce false positives, which means not only reducing the human investment of analysts, but also guaranteeing a better customer experience.
The Products You May Be Interested In
![]() |
3423 | RUGGED METAL PUSHBUTTON | 270 More on Order |
![]() |
3350 | RUGGED METAL PUSHBUTTON | 183 More on Order |
![]() |
559 | RUGGED METAL PUSHBUTTON | 396 More on Order |
![]() |
481 | RUGGED METAL PUSHBUTTON | 499 More on Order |
![]() |
3984 | PLATINUM RTD SENSOR - PT1000 - 3 | 414 More on Order |
![]() |
381 | WATERPROOF DS18B20 DIGITAL TEMPE | 1191 More on Order |
![]() |
952 | WIRE EL FLOW EFFECT INV GREEN 2M | 490 More on Order |
![]() |
2736 | ADDRESS LED MATRIX SERIAL RGB | 473 More on Order |
![]() |
2551 | NEOPIXEL DIGITAL RGB LED STRIP - | 424 More on Order |
![]() |
2873 | ADDRESS LED RING 1/4 SER RGBW | 462 More on Order |
![]() |
2863 | ADDRESS LED RING SERIAL RGBW | 119 More on Order |
![]() |
2870 | ADDRESS LED MATRIX SERIAL RGBW | 320 More on Order |
![]() |
3729 | ADDRESS LED MODULE SERIAL RGB | 343 More on Order |
![]() |
2828 | ADDRESS LED STRIP SERIAL RGBW 1M | 579 More on Order |
![]() |
872 | ADDRESS LED MATRIX I2C GREEN | 293 More on Order |
![]() |
2848 | ADDRESS LED STRIP SERIAL RGBW 1M | 244 More on Order |
![]() |
3630 | ADDRESS LED STRIP RGBW | 325 More on Order |
![]() |
3444 | ADDRESS LED MATRIX SERIAL RGB | 464 More on Order |
![]() |
1507 | ADDRESS LED STRIP SERIAL RGB 1M | 437 More on Order |
![]() |
3860 | FLEXIBLE SILICONE LED STRIP - 1 | 432 More on Order |
![]() |
1934 | 7"" TFT DISPLAY 800 X 480 | 142 More on Order |
![]() |
1743 | 3.2"" TFT LCD WITH TOUCHSCREEN BR | 356 More on Order |
![]() |
338 | LCD KIT NOKIA 5110/3310 MONOCHR | 469 More on Order |
![]() |
1921 | LED BAR 10-SEGMENT RED | 230 More on Order |