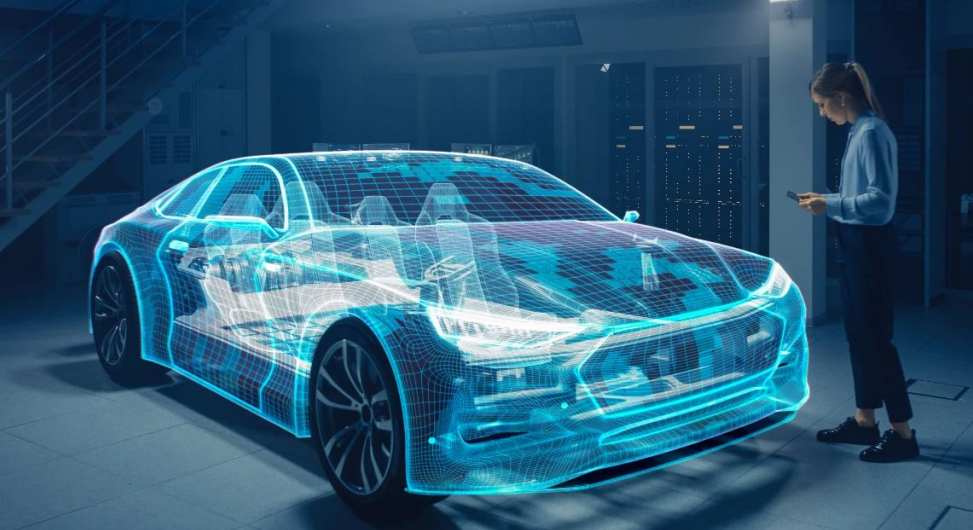
Ai is playing a key role in reshaping the engineering paradigm, providing tools and methods that improve precision, efficiency, and adaptability in various fields. Engineering leaders who want to stay ahead in the AI race should focus on advances in four key areas: generative AI, verification and validation, reduced order model (ROM), and control system design.
Trend 1: GenAI moves to block diagrams, 3D models, and flow charts
While the initial focus on text-based GenAI continues to impact software-centric workflows, its impact on engineering tools with higher levels of abstraction has lagged. Through 2025, we expect GenAI to continue to make progress in the application of "no code" engineering tools such as block diagrams, 3D models, and flow charts. These tools enable engineers to graphically represent complex systems, effortlessly edit components, and manage inherent complexity. In addition, they are critical to engineer productivity and validate the engineer's confidence in system-level performance. Combining GenAI with these tools will further increase their productivity while maintaining an interface familiar to end users. More tools in the field will integrate AI assistants, enabling them to understand engineering models and assist with their design and management.
Trend two: Engineers use verification and validation to achieve AI compliance
As the convergence of AI with safety-critical systems in automotive, healthcare and aerospace applications accelerates, industry regulators are rolling out requirements, frameworks and guidance for AI compliance. In response, engineers must prioritize the verification and validation (V&V) process now to ensure that their AI components are ready to deploy under any conditions and meet potential reliability, transparency, and deviation compliance standards.
V&V is critical for validating the robustness of deep learning models and detecting out-of-distribution (OOD) scenarios, especially in safety-critical applications. Robustness verification is critical because neural networks can misclassify inputs with small, imperceptible changes called adversarial examples. For example, a subtle disturbance in a chest X-ray image may cause the model to incorrectly identify pneumonia as normal. Engineers can provide mathematical proofs of model consistency and test these scenarios using formal verification methods, such as abstract interpretation. This process enhances the reliability of the model and ensures compliance with security standards by identifying and addressing vulnerabilities.
Out-of-distribution detection is equally important because it enables AI systems to recognize and appropriately process unfamiliar inputs. This ability is critical to maintaining accuracy and safety, especially when unexpected data leads to incorrect predictions. The ability to distinguish between in-distribution and out-of-distribution data ensures that AI models can hand uncertain situations over to human experts, preventing potential failures in critical applications.
Focusing on V&V allows engineers to comply with AI frameworks and standards while driving product development within their industry. A proactive compliance approach ensures that AI systems are reliable, safe, and ethical to maintain a competitive advantage in a rapidly evolving environment.
Trend 3: The rise of artificial Intelligence-based reduced order models in engineering
With advances in AI technology and computing power, the trend to use AI-based reduced order models (ROMs) is expected to grow. Engineers using these models will improve system performance and reliability, as well as the efficiency and efficacy of system design and simulation.
The main driver behind this shift is the need for engineers to manage increasingly complex systems while maintaining high accuracy and speed. Traditional computer aided engineering (CAE) and computational fluid dynamics (CFD) models, while accurate, are computationally heavy and not suitable for real-time applications. Ai-based ROMs solve this problem by reducing computational requirements while maintaining accuracy. Engineers can use these models to simulate complex phenomena more quickly, allowing for faster iteration and optimization.
In addition, AI-based ROMs have a highly versatile ability to adapt to different parameters and conditions, enhancing their applicability in different scenarios. This adaptability is particularly valuable in the aerospace, automotive, and energy sectors, where engineered systems often involve complex physical phenomena that require detailed modeling and simulation. For example, engineers designing and testing aircraft components, such as wings or engines, can more effectively simulate aerodynamic characteristics and stress factors, helping engineers iterate and optimize designs quickly. In addition, the AI-based ROM can be adapted to a variety of flight conditions, making it a versatile tool for testing multiple scenarios using the same model. This capability speeds up the development process, reduces costs, and improves the reliability of the final product.
Trend 4: AI breaks down barriers to control of complex systems
The continued convergence of AI and control design will transform the field, especially in managing complex systems and embedded applications. Traditionally, control system design has relied on first-principles modeling, which requires a wealth of knowledge and in-depth understanding of the system. Data-driven modeling is limited to linear models that are valid in a small part of the design scope. Artificial intelligence is changing that by creating accurate nonlinear models from data. This makes it possible to create high-precision models that combine first principles and data and are effective across the entire range of operations. This advance allows for better control of complex systems.
At the same time, the increasing computing power of microcontrollers also promotes the direct embedding of AI algorithms into systems. This integration is particularly influential in the consumer electronics and automotive industries, where highly responsive systems are becoming the norm. For example, AI is embedded in power tools to monitor and respond to environmental changes, such as sudden material density changes that could pose safety risks. These tools use embedded artificial intelligence to autonomously adjust their operations to improve safety and performance.
The convergence of artificial intelligence with complex system control and embedded systems has ushered in an era of more robust, adaptive and intelligent control design. Engineers can now create systems that learn and adapt in real time, providing unprecedented precision and efficiency. This creates an environment in which AI-driven solutions solve traditional control problems and paves the way for smarter, more integrated systems across various engineering domains.
Engineers should be excited about the continued maturation and advancement of AI. The fusion of physical insights with AI models will enhance transparency and adaptability, reducing the "black box" nature of traditional approaches. The proliferation of AI tools has made it easier for engineers to access and use advanced features. These advances will elevate the role of AI in engineering and enable technology professionals to build better engineering systems faster and more efficiently.
The Products You May Be Interested In
![]() |
EP1600-UTEZ | AC/DC CONVERTER 52V 1600W | 104 More on Order |
![]() |
PNDT012A0X43-SRZ | MODULE DC DC CONVERTER | 257 More on Order |
![]() |
UNVT012A0X43-SRZ | MODULE DC DC CONVERTER | 459 More on Order |
![]() |
EHW007A0B41-SZ | DC DC CONVERTER 12V 84W | 247 More on Order |
![]() |
AXA010A0A93-SR | DC DC CONVERTER 10V 50W | 263 More on Order |
![]() |
APXH020A0X3-SRZ | DC DC CONVERTER 0.6-3.63V 73W | 240 More on Order |
![]() |
APTS050A0X43-SRPHZ | DC DC CONVERTER 0.7-2V 100W | 141 More on Order |
![]() |
QW015A0F61 | DC DC CONVERTER 3.3V 50W | 183 More on Order |
![]() |
QRW040A0P41 | DC DC CONVERTER 1.2V 48W | 479 More on Order |
![]() |
QRW025A0Y | DC DC CONVERTER 1.8V 45W | 390 More on Order |
![]() |
QHW100A71 | DC DC CONVERTER 5V 100W | 178 More on Order |
![]() |
NH020F2 | DC DC CONVERTER 3.3V 20W | 382 More on Order |
![]() |
MW005AJ | DC DC CONVERTER +/-5V 5W | 454 More on Order |
![]() |
LW025A1 | DC DC CONVERTER 5V 25W | 239 More on Order |
![]() |
LW020A7 | DC DC CONVERTER 5V 20W | 252 More on Order |
![]() |
HW100F1 | DC DC CONVERTER 3.3V 66W | 163 More on Order |
![]() |
ATH006A0X | DC DC CONVERTER 0.8-3.6V 21W | 370 More on Order |
![]() |
QBVS050A0B61-HZ | DC DC CONVERTER 12V 600W | 447 More on Order |
![]() |
QBVW033A0B841-PZ | DC DC CONVERTER 12V 400W | 434 More on Order |
![]() |
JRCW450R541Z | DC DC CONVERTER 32V 450W | 247 More on Order |
![]() |
QSVW035A0B41Z | DC DC CONVERTER 12V 420W | 125 More on Order |
![]() |
ESTW025A0F41Z | DC DC CONVERTER 3.3V 83W | 445 More on Order |
![]() |
ESTW015A0F641Z | DC DC CONVERTER 5V 75W | 160 More on Order |
![]() |
SSTW003A0A41-SRZ | DC DC CONVERTER 5V 15W | 450 More on Order |