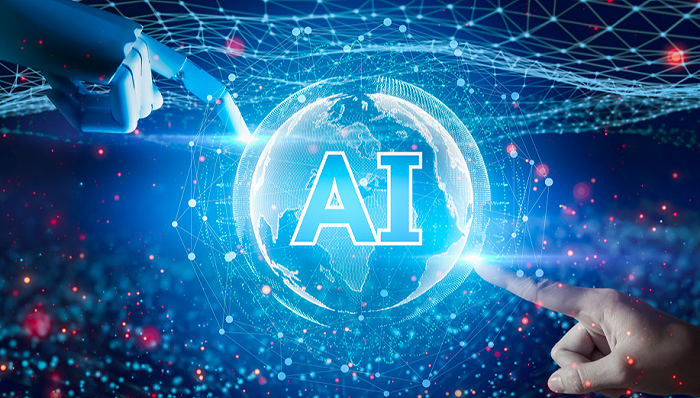
Image processing is a process that converts digital images into other forms or extracts the features of images. Artificial intelligence and machine learning techniques are widely used in image processing, and they can help us identify, analyze and process images more accurately. This paper will introduce the image processing technology based on artificial intelligence and machine learning.
Ⅰ, Image processing based on artificial intelligence
Ai IRF640NPBF is a computer technology that mimics human intelligence to help us better understand and process images. The following are the image processing techniques based on artificial intelligence.
1. Image classification
Image classification refers to the classification of images into different categories. This technology can be used to identify objects or scenes in images. Artificial intelligence techniques can be used to classify images by training neural networks. Deep learning algorithms such as convolutional neural networks (CNN) and recurrent neural networks (RNN) are widely used in image classification tasks.
2. Target detection
Object detection refers to finding one or more specific objects in an image. This technology can be used for autonomous driving, security monitoring and other fields. Ai-based object detection techniques include deep learning-based methods and traditional computer vision methods.
3. Image segmentation
Image segmentation refers to dividing an image into different parts or regions. This technology can be used in medical image analysis, remote sensing image analysis and other fields. Artificial Intelligence-based image segmentation techniques include deep learning-based methods and traditional computer vision methods.
4. Image enhancement
Image enhancement refers to the improvement of image quality and sharpness through a series of processing techniques. This technology can be used for image reconstruction, video compression and other fields. Ai-based image enhancement techniques include deep learning-based methods and traditional computer vision methods.
Ⅱ, Image processing based on machine learning
Machine learning is a technique that allows computers to learn and improve from data. It can help us understand and process images better. The following are image processing techniques based on machine learning.
1. Feature extraction
Feature extraction refers to extracting useful information from images. This technology can be used in image classification, target detection and other fields. Machine learning algorithms such as support vector machines (SVM) and random forests (RF) are widely used in image feature extraction tasks.
2. Image classification
Image classification refers to the classification of images into different categories. This technology can be used to identify objects or scenes in images. Machine learning algorithms such as SVM and RF are widely used in image classification tasks.
3. Target detection
Object detection refers to finding one or more specific objects in an image. This technology can be used for autonomous driving, security monitoring and other fields. Machine learning algorithms such as convolutional neural networks (CNN) and recurrent neural networks (RNN) are widely used in image object detection tasks.
4. Image segmentation
Image segmentation refers to dividing an image into different parts or regions. This technology can be used in medical image analysis, remote sensing image analysis and other fields. Machine learning algorithms such as clustering and random forest are widely used in image segmentation tasks.
Ⅲ, Image processing based on artificial intelligence and machine learning
Image processing technology based on artificial intelligence and machine learning combines the advantages of the two technologies to better process and analyze images. The following are image processing techniques based on artificial intelligence and machine learning.
1. Image denoising
Image denoising is the removal of noise from the image. Artificial intelligence and machine learning techniques are widely used in image denoising tasks. Deep learning algorithms such as autoencoders (AE) and generative adversarial networks (Gans) are widely used in image denoising tasks.
2, image super resolution
Image super resolution refers to the improvement of image resolution through a series of processing techniques. Artificial intelligence and machine learning techniques are widely used in image super-resolution tasks. Deep learning algorithms such as convolutional neural networks (CNN) and recurrent neural networks (RNN) are widely used in image super-resolution tasks.
3. Image generation
Image generation refers to the generation of new images through a series of processing techniques. Artificial intelligence and machine learning techniques are widely used in image generation tasks. Deep learning algorithms such as GAN and variational autoencoders (VAE) are widely used in image generation tasks.
4. Image analysis
Image analysis refers to the analysis of information in images through a series of processing techniques. Artificial intelligence and machine learning techniques are widely used in image analysis tasks. Deep learning algorithms such as CNNS and RNNS are widely used in image analysis tasks.
In summary, artificial intelligence and machine learning techniques have been widely used in image processing, and they can help us identify, analyze and process images more accurately. In the future, with the continuous development of artificial intelligence and machine learning technologies, they will play an increasingly important role in the field of image processing.
The Products You May Be Interested In
![]() |
TPCM-2.4-5 | CMC 2.41MH 5A 2LN TH | 383 More on Order |
![]() |
62054-P2S02 | XFRMR TOROIDAL 15VA CHAS MOUNT | 107 More on Order |
![]() |
62051-P2S02 | XFRMR TOROIDAL 15VA CHAS MOUNT | 184 More on Order |
![]() |
62045-P2S02 | XFRMR TOROIDAL 10VA CHAS MOUNT | 135 More on Order |
![]() |
62035-P2S02 | XFRMR TOROIDAL 7VA CHAS MOUNT | 369 More on Order |
![]() |
62034-P2S02 | XFRMR TOROIDAL 7VA CHAS MOUNT | 441 More on Order |
![]() |
62025-P2S02 | XFRMR TOROIDAL 5VA CHAS MOUNT | 218 More on Order |
![]() |
62075-P2S02 | XFRMR TOROIDAL 35VA CHAS MOUNT | 406 More on Order |
![]() |
62073-P2S02 | XFRMR TOROIDAL 35VA CHAS MOUNT | 294 More on Order |
![]() |
70072K | XFRMR TOROIDAL 35VA THRU HOLE | 313 More on Order |
![]() |
70054K | XFRMR TOROIDAL 15VA THRU HOLE | 262 More on Order |
![]() |
70041K | XFRMR TOROIDAL 10VA THRU HOLE | 157 More on Order |
![]() |
70031K | XFRMR TOROIDAL 7VA THRU HOLE | 481 More on Order |
![]() |
70024K | XFRMR TOROIDAL 5VA THRU HOLE | 486 More on Order |
![]() |
70014K | XFRMR TOROIDAL 3.2VA THRU HOLE | 309 More on Order |
![]() |
70013K | XFRMR TOROIDAL 3.2VA THRU HOLE | 244 More on Order |
![]() |
70002K | XFRMR TOROIDAL 1.6VA THRU HOLE | 280 More on Order |
![]() |
62015-P2S02 | XFRMR TOROIDAL 3.2VA CHAS MOUNT | 210 More on Order |
![]() |
70025K | XFRMR TOROIDAL 5VA THRU HOLE | 226 More on Order |
![]() |
62082-P2S02 | XFRMR TOROIDAL 50VA CHAS MOUNT | 277 More on Order |
![]() |
62033-P2S02 | XFRMR TOROIDAL 7VA CHAS MOUNT | 360 More on Order |
![]() |
62084-P2S02 | XFRMR TOROIDAL 50VA CHAS MOUNT | 607 More on Order |
![]() |
70034K | XFRMR TOROIDAL 7VA THRU HOLE | 674 More on Order |
![]() |
AC1025 | TRANSFORMER CURRENT 25.0 AMP | 235 More on Order |