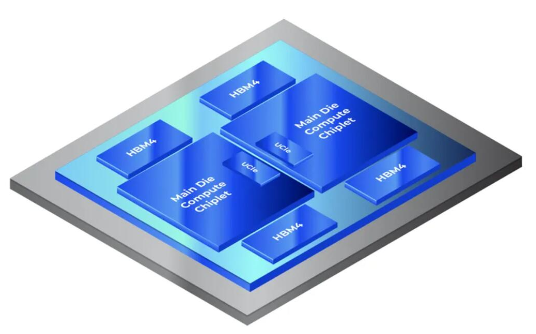
Artificial intelligence (AI) and machine learning (ML) are advancing at an extraordinary pace, powering progress across industries. As models become larger and more complex, they need to process large amounts of data in real time. This demand puts pressure on the underlying hardware infrastructure, especially memory, which must process massive data sets quickly and efficiently. High-bandwidth memory (HBM) has emerged as a key enabler for a new generation of AI, providing the capacity and performance needed to push the boundaries of what AI can achieve.
The latest leap in HBM technology, HBM4, is expected to further enhance AI systems. With enhanced memory bandwidth, higher efficiency, and advanced design, HBM4 will be the backbone of future AI advancements, especially in large-scale data-intensive applications such as natural language processing, computer vision, and autonomous systems.
The need for advanced memory in AI systems
AI workloads, particularly deep neural networks, differ from traditional computing in that they require processing large data sets in parallel, which presents unique memory challenges. These models require high data throughput and low latency for optimal performance. High Bandwidth Memory (HBM) addresses these needs by providing superior bandwidth and energy efficiency. Unlike traditional memory, which uses a wide external bus, HBM's vertically stacked chips and direct processor interface minimize data transmission distances, enabling faster transfers and reducing power consumption, making it ideal for high-performance AI systems.
How does the HBM4 improve on previous generations
HBM4 significantly improves AI and ML performance by increasing bandwidth and memory density. HBM4 has higher data throughput, enabling AI accelerators and Gpus to more efficiently process hundreds of gigabits per second, reducing bottlenecks and improving system performance. It achieves higher memory density by adding more layers to each stack, solving the huge storage requirements of large AI models and facilitating smoother scaling of AI systems.
Energy efficiency and scalability
As AI systems continue to expand, energy efficiency is becoming an increasing concern. AI training models are very power-hungry, and as data centers expand their AI capabilities, the need for energy-efficient hardware becomes critical. The HBM4 was designed with energy efficiency in mind. Its stacked architecture not only shortens data transmission distances, but also reduces the power consumption required to move data. The HBM4 achieves better performance per watt compared to previous generations, which is critical for the sustainability of large-scale AI deployments.
Scalability is another highlight of HBM4. The ability to stack multiple layers of memory while maintaining high performance and low power consumption means AI systems can scale without becoming too expensive or inefficient. As AI applications expand from dedicated data centers to edge computing environments, scalable memory like the HBM4 becomes critical to deploying AI in a variety of use cases, from self-driving cars to real-time language translation systems.
Optimize AI hardware with HBM4
Integrating HBM4 into AI hardware is critical to unlocking the full potential of modern AI accelerators, such as Gpus and custom AI chips, which require low-latency, high-bandwidth memory to support massively parallel processing. HBM4 improves reasoning speed, which is critical for real-time applications such as autonomous driving, and accelerates AI model training by providing higher data throughput and greater memory capacity. These advancements enable faster and more efficient AI development, allowing for faster model training and improved performance of AI workloads.
The role of HBM4 in large language models
HBM4 is ideal for developing large language models (LLMS) like GPT-4 that drive generative AI applications such as natural language understanding and content generation. LLM requires a large amount of memory resources to store billions or trillions of parameters and handle data processing efficiently. HBM4's high capacity and bandwidth enable rapid access and transmission of data needed for inference and training, support increasingly complex models, and enhance AI's ability to generate human-like text and solve complex tasks.
Conclusion
The Products You May Be Interested In
![]() |
CP3000AC54TEPZ-F | AC/DC CONVERTER | 120 More on Order |
![]() |
GP100H3R48TEZ | AC/DC CONVERTER 52V 6000W | 466 More on Order |
![]() |
PNVT012A0X43-SRZ | MODULE DC DC CONVERTER | 471 More on Order |
![]() |
HW010A0F1-SR | DC DC CONVERTER 3.3V 33W | 358 More on Order |
![]() |
SW003A0A94-SRZ | DC DC CONVERTER 5V 15W | 292 More on Order |
![]() |
EHHD020A0F41-SZ | DC DC CONVERTER 3.3V 66W | 215 More on Order |
![]() |
HW010A0F1-SZ | DC DC CONVERTER 3.3V 33W | 156 More on Order |
![]() |
EQW020A0A41-HZ | DC DC CONVERTER 5V 100W | 138 More on Order |
![]() |
QPW060A0M1 | DC DC CONVERTER 1.5V 90W | 274 More on Order |
![]() |
QHW075G71 | DC DC CONVERTER 2.5V 38W | 272 More on Order |
![]() |
MC005BK | DC DC CONVERTER +/-12V 5W | 282 More on Order |
![]() |
LW015A84 | DC DC CONVERTER 5V 15W | 415 More on Order |
![]() |
JW150G1 | DC DC CONVERTER 2.5V 75W | 118 More on Order |
![]() |
JC100B1 | DC DC CONVERTER 12V 100W | 107 More on Order |
![]() |
QBVW025A0B1-PHZ | DC DC CONVERTER 12V 300W | 365 More on Order |
![]() |
JNCW016A0R41Z | DC DC CONVERTER 28V 448W | 120 More on Order |
![]() |
QBVW025A0B1Z | DC DC CONVERTER 12V 300W | 469 More on Order |
![]() |
ERCW003A6R41-HZ | DC DC CONVERTER 28V | 295 More on Order |
![]() |
EHHD020A0F641Z | DC DC CONVERTER 3.3V 66W | 338 More on Order |
![]() |
EHHD006A0B641Z | DC DC CONVERTER 12V 72W | 354 More on Order |
![]() |
ESTW004A2C841Z | DC DC CONVERTER 15V 63W | 404 More on Order |
![]() |
ATH030A0X3-SRZ | DC DC CONVERTER 0.8-3.63V 109W | 311 More on Order |
![]() |
ATH016A0X3-SRZ | DC DC CONVERTER 0.8-3.6V 58W | 146 More on Order |
![]() |
TJT120A0X43-SZ | DC DC CONVERTER 0.6-1.5V | 351 More on Order |