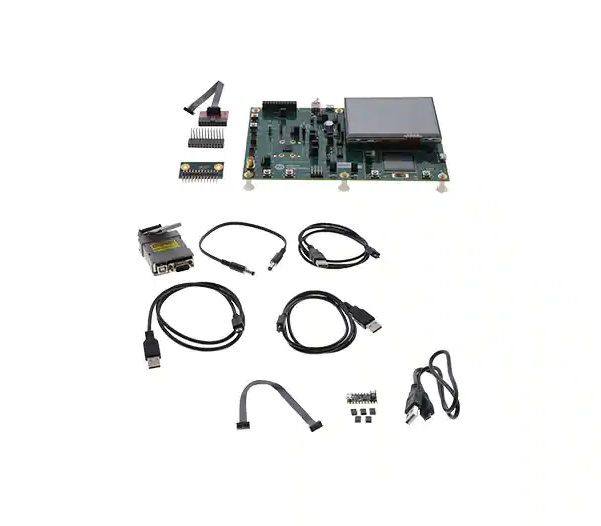
In general, edge AI is relative to cloud AI in other words, it allows devices to do AI locally before data processing, computation and decision making do not necessarily need to be connected to the Internet.
Compared with cloud AI, edge AI has many characteristics such as good real-time performance, low bandwidth resource requirements, and good privacy. Therefore, in many scenarios, edge AI is better at solving real problems than cloud AI.
Edge AI is suitable for scenarios with high time sensitivity
For example, a self-driving car suddenly finds a child in the road ahead. To avoid disaster, cars must react within milliseconds. If the data is uploaded to the cloud, there will be a large delay, so the problem of edge AI can be solved.
Another example is forest fire monitoring, highway, railway or dam landslide monitoring. These places are often remote and often do not have good communication networks, but quick decision making and early warning are critical, so the edge ends must be identified quickly.
For scenarios where application time is very sensitive, traditional cloud monitoring may require sending a set of pictures to the cloud, which has a high demand on network traffic. Through the independent intelligent recognition of the monitoring side, only when the situation occurs to send a short message, in order to quickly realize the safety warning, to avoid more serious disaster losses.
Like cloud AI, edge AI also has AI common characteristics. AI technology is a powerful ADS826E pattern recognition technology that requires a lot of matrix operations and requires a lot of storage space and system power consumption. AI performs AI locally on the device, and the battery usually needs to be powered, so there is a need to balance system power consumption, computing speed, and device cost.
However, low-power microcontrollers need to call a lot of resources to perform AI operations, and the speed is low, which can only be simple calculations. FPGA, GPU Although some large processors compute fast, but high cost, high power consumption; Low-cost microcontrollers/microprocessors have poor performance in terms of power consumption characteristics, and the computing speed and computational complexity are also average.
ADI low power microcontroller with neural network accelerator
The new generation of solutions requires more ultra-low power characteristics. ADI's MAX78000 low-power microcontroller with a neural network accelerator delivers an optimal balance of power consumption, speed and cost.
Specifically, the MAX78000 integrates two MCU system control cores, the Arm Cortex-M4 processor and the 32-bit RISC-V, where the processor Arm Cortex-M4F processor is 100MHz and customers can write any system management code. The function of the RISC-V processor is to quickly load data into the neural network accelerator with low power consumption, and users can use any microcontroller core to input data into the convolutional neural network engine.
The MAX78000 has 432KB compared to software solutions running on low-power microcontrollers, after configuring and loading data, the MAX78000 runs AI inference 100 times faster with less than 1% power consumption.
ADI Senior business Manager Yong Li previously introduced that low power consumption is a key requirement for many edge intelligence application scenarios, and the MAX78000 in addition to being based on the CNN accelerator and dual-core processor architecture also provides efficient internal power management, integrated single inductor output (SIMO) switching mode power supply, to maximize the battery power range of iot devices.
According to Yi Xin, an application engineer at ADI, the MAX's most unique feature is that the 78000CNN accelerator is a special piece of hardware designed to maximize the operational energy consumption and latency of the geographic neural network. The CNN Accelerator has 432KB weight storage to support up to 3.5 million weight networks, and the product architecture is highly flexible, allowing TensorFlow, PyTorch regular toolset training, and running on the MAX78000 after conversion.
Another feature of the MAX78000 is that there is less MCU intervention, and during the operation of the CNN, the accelerator is almost free from any interference affecting the MCU core, which greatly simplifies the operation, improves efficiency, and improves latency.
Due to superior design, the power consumption of the chip is greatly reduced, allowing the chip to perform complex power AI in battery-powered devices while the application does not cause delays and cost increases.
Compared with the above software solutions, the MAX78000 executes AI 100 times faster and costs only 100 times the FPGA solution or the GPU zero-end MCU+DSP solution, and the MAX78000 consumes less than 1% power. The MAX78000 achieves the optimal balance of power consumption, speed and cost.
MAX78000 has been widely used in forest fire prevention monitoring, geological disaster monitoring and smart home. Li said that many similar applications that do not involve public safety also need to enable local fast intelligent decision-making. For example, some users want to wear a camera while riding a bike, achieve voice control anytime, anywhere and develop products, similar to voice control of hearing AIDS, predictive maintenance of wind power equipment, etc.
The introduction of this edge intelligence solution based on ultra-low power hardware computing power, high integration, small size and low cost provides a truly viable intelligent authorization solution for more and more application areas.
The Products You May Be Interested In
![]() |
AMI-22B-60-6A | LINE FILTER 250VAC 60A CHASS MNT | 450 More on Order |
![]() |
AMI-M12Z-6-20-B | LINE FILTER 250VAC 6A CHASS MNT | 136 More on Order |
![]() |
AMI-M11F-3-3-A | LINE FILTER 250VAC 3A CHASS MNT | 225 More on Order |
![]() |
AMI-28B-12-1 | LINE FILTER 110/250VAC 12A CHAS | 128 More on Order |
![]() |
AMI-27A-16-1 | LINE FILTER 250VAC 16A CHASS MNT | 296 More on Order |
![]() |
AMI-26B-12-1 | LINE FILTER 250VAC 12A CHASS MNT | 396 More on Order |
![]() |
AMI-M12R-3-10-B-2 | LINE FILTER 250VAC 3A CHASS MNT | 288 More on Order |
![]() |
AMI-M12S-7M-5-B-2 | LINE FILTER 250VAC 7A CHASS MNT | 288 More on Order |
![]() |
AMI-23B-10-3 | LINE FILTER 250VAC 10A CHASS MNT | 102 More on Order |
![]() |
AMI-M11K-7M-5-B-1 | LINE FILTER 250VAC 7A CHASS MNT | 364 More on Order |
![]() |
AMI-M11D-3-6-B-2 | LINE FILTER 250VAC 3A CHASS MNT | 231 More on Order |
![]() |
AMI-22A-12-1 | LINE FILTER 250VAC 12A CHASS MNT | 371 More on Order |
![]() |
AMI-M11UF-3-16-B | LINE FILTER 250VAC 3A CHASS MNT | 384 More on Order |
![]() |
AMI-29-20-6 | LINE FILTER 110/250VAC 20A CHASS | 166 More on Order |
![]() |
AMI-27-10-1 | LINE FILTER 110/250VAC 10A CHASS | 260 More on Order |
![]() |
AMI-M12AB-6-20-B | LINE FILTER 250VAC 20A CHASS MNT | 371 More on Order |
![]() |
AMI-29A-12-1 | LINE FILTER 110/250VAC 12A CHASS | 241 More on Order |
![]() |
AMI-M12B-6-20-C | LINE FILTER 250VAC 20A CHASS MNT | 368 More on Order |
![]() |
AMI-27B-3-1 | LINE FILTER 110/250VAC 3A CHAS | 202 More on Order |
![]() |
AMI-29A-6-1 | LINE FILTER 110/250VAC 6A CHAS | 389 More on Order |
![]() |
AMI-M12W-1-10-C | LINE FILTER 250VAC 10A CHASS MNT | 100 More on Order |
![]() |
AMI-21-12-1 | LINE FILTER 250VAC 12A CHASS MNT | 365 More on Order |
![]() |
AMI-22-12-1 | LINE FILTER 250VAC 12A CHASS MNT | 333 More on Order |
![]() |
AMI-21B-10-1 | LINE FILTER 250VAC 10A CHASS MNT | 130 More on Order |