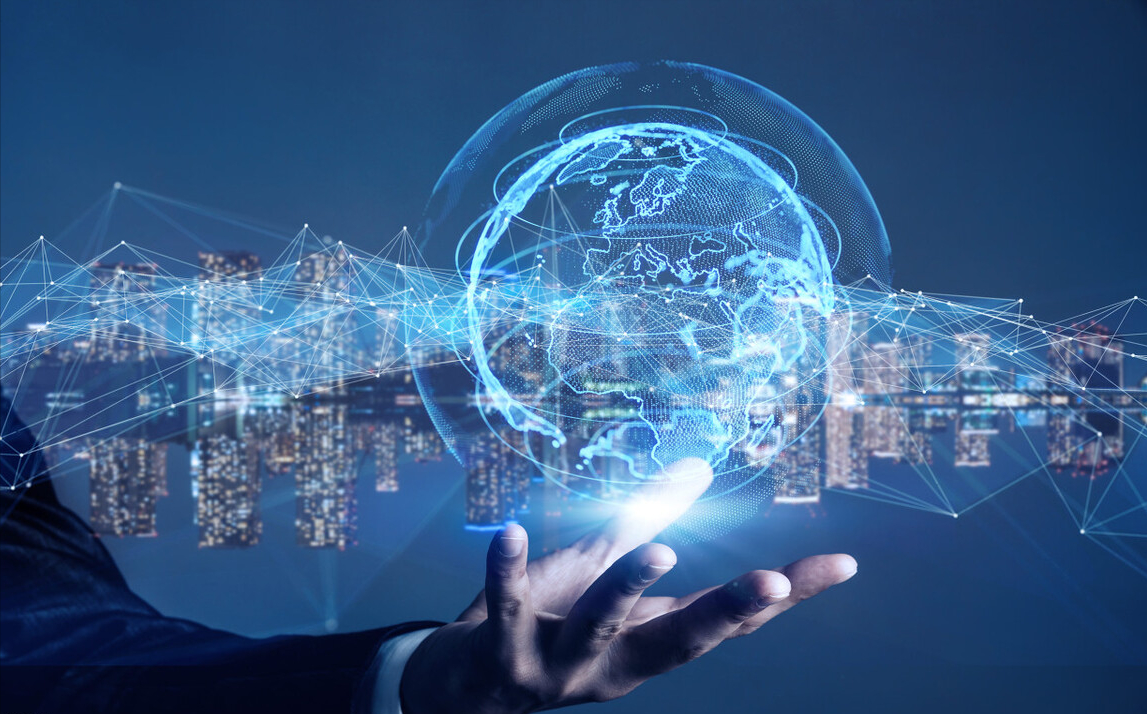
With the popularity of the Internet of Things and the next generation of smart devices, low-power electronic devices and chips are increasingly finding their way into millions of homes, and low-power design is becoming increasingly important. On the one hand, the Internet of Things and smart devices limit the size of devices, on the other hand, they have high cost requirements. In addition, in some usage scenarios, the battery replacement and charging cycle is required (for example, the battery replacement cycle is monthly or longer), so there is a high limit on the battery capacity, which requires that the chip can use a low-power design.
On the other hand, AI features are increasingly being added to the Internet of Things and smart devices. Ai can provide important new features for the Internet of Things and smart devices. For example, voice AI can provide wakeword recognition, voice command recognition, etc. Machine vision AI can provide face detection, event detection and so on. As mentioned earlier, the Internet of Things and smart devices require low power consumption, so incorporating AI features also requires low power AI.
Nowadays, AI chips have been popularized in the cloud and intelligent device terminals, such as Nvidia and cloud AMDGPU, represented by GPU. Intel/Habana is represented by artificial intelligence acceleration chip; In terminal smart devices, AI is mainly accelerating IP on SoC, but whether it is GPU or GPUSoC AI, the world is accelerating IP because the need for low power is not taken into account, so future iot and AI in smart devices will require new low power related designs.
Sensor Computing: An important technology path for low power AI
In smart devices and iot applications, low-power AI needs to reduce power consumption to very low levels to enable real-time online (always-on) AI services. This is called always-on, which means that the AI needs to be used all the time before working, rather than being turned on by the user. On the one hand, it is necessary to continuously open the relevant sensor to detect the signal of the relevant mode in real time. On the other hand, AI needs to achieve low power consumption.
In traditional design, the function of the sensor is to collect high-performance signals, and transfer the collected signals to the processor (SoC or MCU) for further calculation and processing, but the sensor itself does not have computing capacity. However, the traditional design assumption is that the associated processor should be turned on at the same time when the sensor is turned on, which does not meet the requirement of alway on AI, because SoC and MCU have a high battery consumption if they are running AI algorithm all the time. On the other hand, from a practical point of view, the always-on AI application mainly hopes that the AI will run all the time so that it can respond in real time when important and relevant events occur (such as IMUs turning off push notifications of smart devices when they detect that the user is driving), but in fact, the frequency of such related events is not very high. If the AI module of SoC or MCU is turned on all the time, the output of AI model will be "undetected event" most of the time.
Combined with these two points, more and more attention is paid to the operation calculation of sensor side. always-on In low-power AI, sensors need to be turned on all the time, no matter what. Therefore, if the sensor has the ability to compute AI, the AI model can do so without turning on the AI module that SoC or MCU runs on the sensor side.
In addition, AI can also avoid sensors and sensors running on the sensor side. SoC/MCU data is transmitted to each other all the time, further reducing power consumption. Finally, sensor-side AI modules can be customized and optimized to best suit the AI algorithm for the sensor, regardless of universality, thus achieving a very high energy efficiency ratio.
Sensor-side AI has its own limitations, of course. On the other hand, in terms of performance and cost, computing and storage space on the sensor side is usually small, and AI modules cannot support large models, so the performance of models is relatively limited. On the other hand, sensor-side AI, as mentioned above, struggles to support generic models, but usually only supports specific operators and model structures.
To sum up, sensor-based AI can achieve low power consumption, but its model performance is relatively limited. On the other hand, in low-power AI scenarios, the relevant events that really need to be processed are not frequent. Combined, sensor-side AI is best suited to running special little models that filter out most irrelevant events; After the sensor-side AI detects relevant events, the sensor can wake up the SoC or MCU to meet the low power consumption, and then confirm the alway on requirement of the upper layer AI.
In the field of image sensor, Sony has launched IMX500 series sensors, which stack sensor chips and logic chips with artificial intelligence computing capabilities, so as to transmit pixel signals to the artificial intelligence computing engine on the logic chip, so that the output of the sensor can be the output of image, artificial intelligence model, or a combination of the two. In this way, the sensor can run always-on at low power and only when its model output meets certain conditions (such as the detection of a face) can the MCU or SoC be awakened for the next action. We expect Sony to further improve its AI capabilities to increase its lead in the next sensor chip segment.
Another example of the combination of sensors and AI is ST's IMU series of products. ST integrates Machine Learning Core and Finite State Machine into the IMUs with relevant AI features, thus supporting AI computing directly on the IMUs in a very efficient way.
Currently, the artificial intelligence algorithm supported by ST is mainly decision tree algorithm, and it can support some important feature extraction of IMU signal (such as signal amplitude, signal variance, etc.), so as to realize user activity classification (such as stationary, walking, cycling, driving, etc.) directly on IMU. This wakes up the MCU/SoC for the next step when an event is detected.
Based on the information published by ST, MLC consumption is on the order of microwatts, so it can support the always-on requirement well. On the other hand, of course, we also see that decision tree algorithms are actually limited in their ability to model complex activities. Thus, as we discussed earlier, the AI in the IMU sensor here is suited to filter out irrelevant events in the first filter of completion events, and more complex classification and validation via MCU or MCUSoC can be done in the running model on top.
The Products You May Be Interested In
![]() |
TPCM-2.4-5 | CMC 2.41MH 5A 2LN TH | 383 More on Order |
![]() |
AA53002-015 | XFRMR TOROIDAL 300VA CHAS MOUNT | 464 More on Order |
![]() |
62054-P2S02 | XFRMR TOROIDAL 15VA CHAS MOUNT | 107 More on Order |
![]() |
62045-P2S02 | XFRMR TOROIDAL 10VA CHAS MOUNT | 135 More on Order |
![]() |
62044-P2S02 | XFRMR TOROIDAL 10VA CHAS MOUNT | 336 More on Order |
![]() |
62043-P2S02 | XFRMR TOROIDAL 10VA CHAS MOUNT | 304 More on Order |
![]() |
62034-P2S02 | XFRMR TOROIDAL 7VA CHAS MOUNT | 441 More on Order |
![]() |
62025-P2S02 | XFRMR TOROIDAL 5VA CHAS MOUNT | 218 More on Order |
![]() |
62012-P2S02 | XFRMR TOROIDAL 3.2VA CHAS MOUNT | 178 More on Order |
![]() |
62075-P2S02 | XFRMR TOROIDAL 35VA CHAS MOUNT | 406 More on Order |
![]() |
62073-P2S02 | XFRMR TOROIDAL 35VA CHAS MOUNT | 294 More on Order |
![]() |
70072K | XFRMR TOROIDAL 35VA THRU HOLE | 313 More on Order |
![]() |
70054K | XFRMR TOROIDAL 15VA THRU HOLE | 262 More on Order |
![]() |
70041K | XFRMR TOROIDAL 10VA THRU HOLE | 157 More on Order |
![]() |
70024K | XFRMR TOROIDAL 5VA THRU HOLE | 486 More on Order |
![]() |
70015K | XFRMR TOROIDAL 3.2VA THRU HOLE | 421 More on Order |
![]() |
70013K | XFRMR TOROIDAL 3.2VA THRU HOLE | 244 More on Order |
![]() |
70003K | XFRMR TOROIDAL 1.6VA THRU HOLE | 140 More on Order |
![]() |
62015-P2S02 | XFRMR TOROIDAL 3.2VA CHAS MOUNT | 210 More on Order |
![]() |
62060-P2S02 | XFRMR TOROIDAL 25VA CHAS MOUNT | 3963 More on Order |
![]() |
70064K | XFRMR TOROIDAL 25VA THRU HOLE | 2293 More on Order |
![]() |
70034K | XFRMR TOROIDAL 7VA THRU HOLE | 674 More on Order |
![]() |
70005K | XFRMR TOROIDAL 1.6VA THRU HOLE | 401 More on Order |
![]() |
AC1050 | CURR SENSE XFMR 50A T/H | 409 More on Order |